How NLP & NLU Work For Semantic Search
Another pair of classes shows how two identical state or process predicates may be placed in sequence to show that the state or process continues past a could-have-been boundary. In example 22 from the Continue-55.3 class, the representation is divided into two phases, each containing the same process predicate. This predicate uses ë because, while the event is divided into two conceptually relevant phases, there is no functional bound between them. Having an unfixed argument order was not usually a problem for the path_rel predicate because of the limitation that one argument must be of a Source or Goal type. Representations for changes of state take a couple of different, but related, forms.
This article aims to give a broad understanding of the Frame Semantic Parsing task in layman terms. Beginning from what is it used for, some terms definitions, and existing models for frame semantic parsing. This article will not contain complete references to definitions, models, and datasets but rather will only contain subjectively important things. The five translators examined in this study have effectively achieved a balance between being faithful to the original text and being easy for readers to accept by utilizing apt vocabulary and providing essential para-textual information.
Approaches: Symbolic, statistical, neural networks
During procedures, doctors can dictate their actions and notes to an app, which produces an accurate transcription. NLP can also scan patient documents to identify patients who would be best suited for certain clinical trials. Natural language processing can help customers book tickets, track orders and even recommend similar products on e-commerce websites. Teams can also use data on customer purchases to inform what types of products to stock up on and when to replenish inventories.
Together is most general, used for co-located items; attached represents adhesion; and mingled indicates that the constituent parts of the items are intermixed to the point that they may not become unmixed. Spend and spend_time mirror one another within sub-domains of money and time, and in fact, this distinction is the critical dividing line between the Consume-66 and Spend_time-104 classes, which contain the same syntactic frames and many of the same verbs. Similar class ramifications hold for inverse predicates like encourage and discourage. The final category of classes, “Other,” included a wide variety of events that had not appeared to fit neatly into our categories, such as perception events, certain complex social interactions, and explicit expressions of aspect.
Semantic Extraction Models
Since there was only a single event variable, any ordering or subinterval information needed to be performed as second-order operations. For example, temporal sequencing was indicated with the second-order predicates, start, during, and end, which were included as arguments of the appropriate first-order predicates. Semantic search brings intelligence to search engines, and natural language processing and understanding are important components. The semantic analysis creates a representation of the meaning of a sentence. But before deep dive into the concept and approaches related to meaning representation, firstly we have to understand the building blocks of the semantic system. Therefore, in semantic analysis with machine learning, computers use Word Sense Disambiguation to determine which meaning is correct in the given context.
This set involves classes that have something to do with employment, roles in an organization, or authority relationships. The representations for the classes in Figure 1 were quite brief and failed to make explicit some of the employment-related inter-class connections that were implicitly available. The above discussion has focused nlp semantics on the identification and encoding of subevent structure for predicative expressions in language. Starting with the view that subevents of a complex event can be modeled as a sequence of states (containing formulae), a dynamic event structure explicitly labels the transitions that move an event from state to state (i.e., programs).
As English translations of The Analects continue to evolve, future translators can further enhance this work by summarizing and supplementing paratextual information, thereby building on the foundations established by their predecessors. By integrating insights from previous translators and leveraging paratextual information, future translators can provide more precise and comprehensive explanations of core concepts and personal names, thus enriching readers’ understanding of these terms. As delineated in Section 2.1, all aberrant outcomes listed in the above table are attributable to pairs of sentences marked with “None,” indicating untranslated sentences.
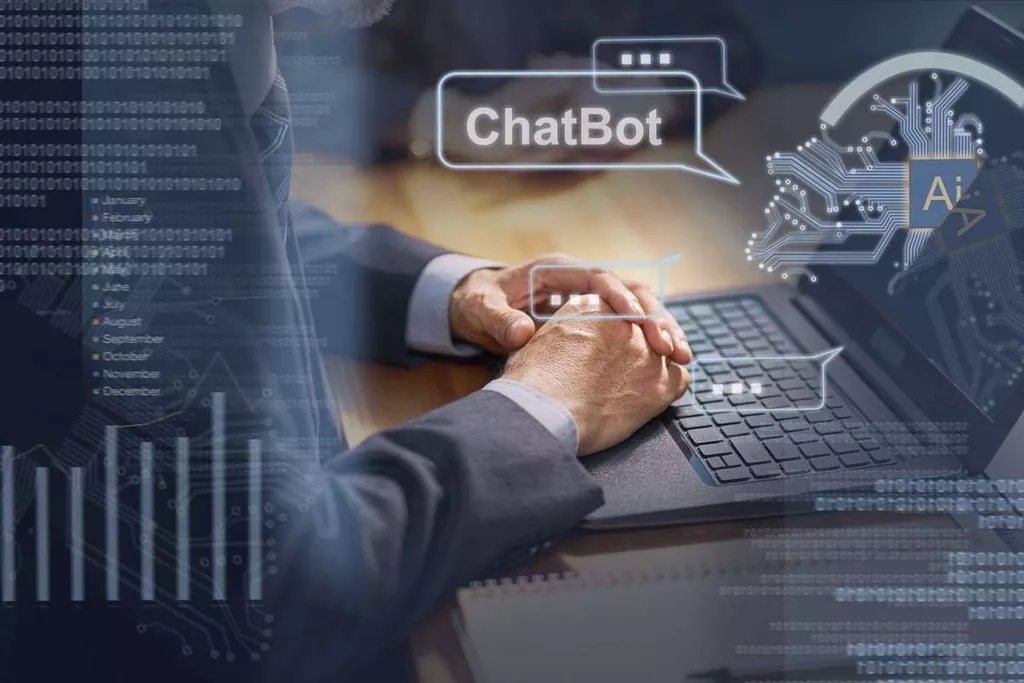
For example, “cows flow supremely” is grammatically valid (subject — verb — adverb) but it doesn’t make any sense. Within the similarity score intervals of 80–85% and 85–90%, the distributions of sentences across all five translators is more balanced, each accounting for about 20%. However, translations by Jennings present fewer instances in the highly similar intervals of 95–100% (1%) and 90–95% (14%). Contrastingly, Slingerland’s translation features a higher percentage of sentences with similarity scores within the 95–100% interval (30%) and the 90–95% interval (24%) compared to the other translators.
Similarly, words like “said,” “master,” “never,” and “words” appear consistently across all five translations. However, despite their recurrent appearance, these words are considered to have minimal practical significance within the scope of our analysis. This is primarily due to their ubiquity and the negligible unique semantic contribution they make. For these reasons, this study excludes these two types of words-stop words and high-frequency yet semantically non-contributing words from our word frequency statistics. 1 represents the computed semantic similarity between any two aligned sentences from the translations, averaged over three algorithms.